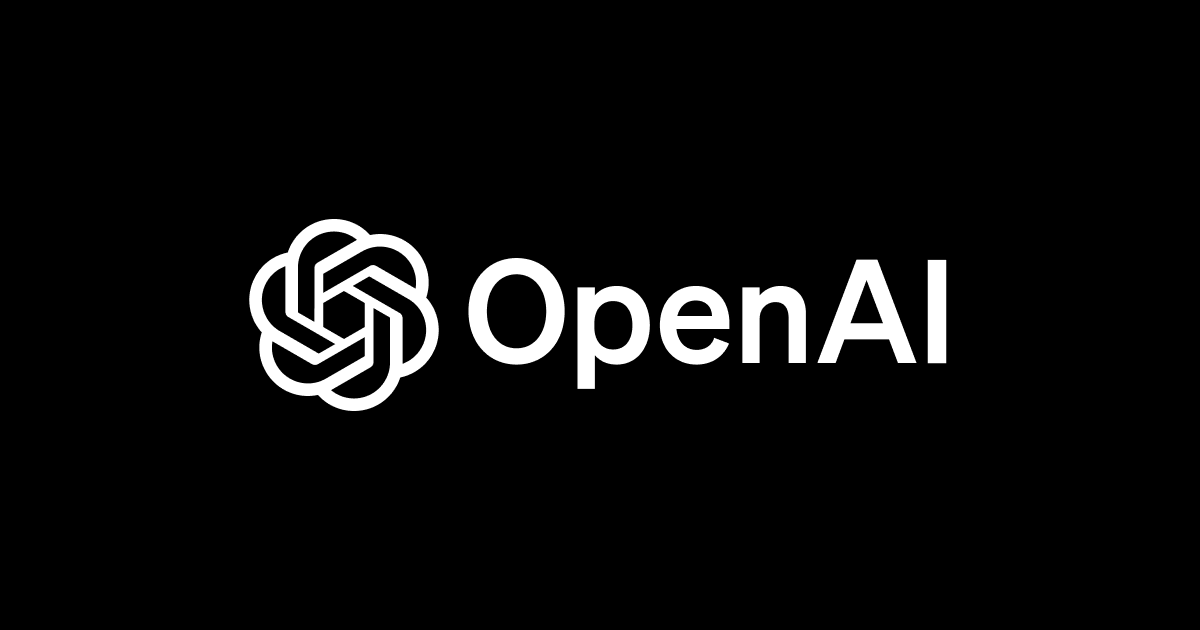
ReRankingを適用したRAGの精度向上について
はじめに
はじめまして クラスメソッド株式会社 新規事業部のレオナです。
クラスメソッド株式会社では、社内情報の検索と回答の精度向上のために、RAG(Retrieval-Augmented Generation)を用いたQAチャットボットを運用と検証しています。このシステムは、ユーザーからの質問に対して関連する社内文書を検索し、LLMがそれらの情報を基に回答を生成します。しかし、運用の中で、ユーザーが常に必要とする情報を得られないという問題があります。
この問題に対処するため、Re-ranking(再ランク)という手法を用いて問題の解決を試みます。Re-rankingは、Retrieverによって取得された複数の文書を、クエリに対する関連度を別ベクトルを用いて高い順別に並べ替える手法です。これによって検索の精度があがり、チャットボットの回答精度が上がることが期待できます。
実装
今回、参考・引用したサンプルコードになります。
- https://github.com/openai/openai-cookbook/blob/main/examples/Search_reranking_with_cross-encoders.ipynb
- https://github.com/aws-samples/amazon-bedrock-rag-workshop/blob/dcdb2f64f796c53a2e226c57447711843e901bca/05_Semantic_Search_with_Reranking/02_LlamaIndex_Reranker_Bedrock_Titan.ipynb
使用するLLMはOpenAIのText-Embedding-Ada-002とAWS BedrockのAmazon.Titan-Embed-Text-v1を使用しています。LLMモデルを使用するにあたって、OpenAIのAPIとAWS Bedrockのモデルの有効化が必要になります。AWS Bedrockの有効化については詳しくはこちらをご覧ください。
Amazon Bedrock をマネジメントコンソールからちょっと触ってみたいときは Base Models(基盤モデル)へのアクセスを設定しましょう
検証では、まず具体的なクエリを定義し、それに関連する文書を用意する必要があります。今回はOpenAIのサンプルコードで書かれている、arxiv(アーカイブ)という査読前論文投稿サイトが提供しているarxiv APIを用いてクエリ検索を行い、論文のAbstractを文書として扱います。
論文情報の取得
1.arxivのライブラリをインポートし、クエリは以下のように定義します。
# pipインストールでarxiv APIが使えるようになります。 pip install arxiv
import arxiv query = "how do bi-encoders work for sentence embeddings" client_arxiv = arxiv.Client() search = arxiv.Search( query=query, max_results=20, sort_by=arxiv.SortCriterion.Relevance )
2.クエリに対する検索結果です。比較する対象として、論文のタイトルのみ表示させます。
1: A Comparative Study of Sentence Embedding Models for Assessing Semantic Variation 2: SBERT studies Meaning Representations: Decomposing Sentence Embeddings into Explainable Semantic Features 3: Are Classes Clusters? 4: Semantic Composition in Visually Grounded Language Models 5: Evaluating the Construct Validity of Text Embeddings with Application to Survey Questions 6: Learning Probabilistic Sentence Representations from Paraphrases 7: Exploiting Twitter as Source of Large Corpora of Weakly Similar Pairs for Semantic Sentence Embeddings 8: How to Probe Sentence Embeddings in Low-Resource Languages: On Structural Design Choices for Probing Task Evaluation 9: Clustering and Network Analysis for the Embedding Spaces of Sentences and Sub-Sentences 10: Vec2Sent: Probing Sentence Embeddings with Natural Language Generation 11: Non-Linguistic Supervision for Contrastive Learning of Sentence Embeddings 12: SentPWNet: A Unified Sentence Pair Weighting Network for Task-specific Sentence Embedding 13: Learning Joint Representations of Videos and Sentences with Web Image Search 14: Character-based Neural Networks for Sentence Pair Modeling 15: Train Once, Test Anywhere: Zero-Shot Learning for Text Classification 16: Efficient Domain Adaptation of Sentence Embeddings Using Adapters 17: Hierarchical GPT with Congruent Transformers for Multi-Sentence Language Models 18: Sentence-T5: Scalable Sentence Encoders from Pre-trained Text-to-Text Models 19: In Search for Linear Relations in Sentence Embedding Spaces 20: Learning to Borrow -- Relation Representation for Without-Mention Entity-Pairs for Knowledge Graph Completion
ソート結果
各LLMモデルがクエリとAbstractに対する関連度の高い順番にソートした結果が以下の通りになりました。各LLMによる再ランク付けされ順序が入れ替わりました。
arxiv オリジナル | AWS Bedrock Amazon.Titan-Embed-Text-v1 | OpenAI Text-Embedding-Ada-002 |
---|---|---|
A Comparative Study of Sentence Embedding Models for Assessing Semantic Variation | A Comparative Study of Sentence Embedding Models for Assessing Semantic Variation | Vec2Sent: Probing Sentence Embeddings with Natural Language Generation |
SBERT studies Meaning Representations: Decomposing Sentence Embeddings into Explainable Semantic Features | Sentence-T5: Scalable Sentence Encoders from Pre-trained Text-to-Text Models | Are Classes Clusters? |
Are Classes Clusters? | In Search for Linear Relations in Sentence Embedding Spaces | Semantic Composition in Visually Grounded Language Models |
Semantic Composition in Visually Grounded Language Models | Sentence-T5: Scalable Sentence Encoders from Pre-trained Text-to-Text Models | Sentence-T5: Scalable Sentence Encoders from Pre-trained Text-to-Text Models |
Evaluating the Construct Validity of Text Embeddings with Application to Survey Questions | Are Classes Clusters? | How to Probe Sentence Embeddings in Low-Resource Languages: On Structural Design Choices for Probing Task Evaluation |
Learning Probabilistic Sentence Representations from Paraphrases | Are Classes Clusters? | SBERT studies Meaning Representations: Decomposing Sentence Embeddings into Explainable Semantic Features |
Exploiting Twitter as Source of Large Corpora of Weakly Similar Pairs for Semantic Sentence Embeddings | SBERT studies Meaning Representations: Decomposing Sentence Embeddings into Explainable Semantic Features | Exploiting Twitter as Source of Large Corpora of Weakly Similar Pairs for Semantic Sentence Embeddings |
How to Probe Sentence Embeddings in Low-Resource Languages: On Structural Design Choices for Probing Task Evaluation | SBERT studies Meaning Representations: Decomposing Sentence Embeddings into Explainable Semantic Features | Train Once, Test Anywhere: Zero-Shot Learning for Text Classification |
Clustering and Network Analysis for the Embedding Spaces of Sentences and Sub-Sentences | Exploiting Twitter as Source of Large Corpora of Weakly Similar Pairs for Semantic Sentence Embeddings | Clustering and Network Analysis for the Embedding Spaces of Sentences and Sub-Sentences |
Vec2Sent: Probing Sentence Embeddings with Natural Language Generation | Vec2Sent: Probing Sentence Embeddings with Natural Language Generation | A Comparative Study of Sentence Embedding Models for Assessing Semantic Variation |
Non-Linguistic Supervision for Contrastive Learning of Sentence Embeddings | Exploiting Twitter as Source of Large Corpora of Weakly Similar Pairs for Semantic Sentence Embeddings | Efficient Domain Adaptation of Sentence Embeddings Using Adapters |
SentPWNet: A Unified Sentence Pair Weighting Network for Task-specific Sentence Embedding | Semantic Composition in Visually Grounded Language Models | Learning Probabilistic Sentence Representations from Paraphrases |
Learning Joint Representations of Videos and Sentences with Web Image Search | Semantic Composition in Visually Grounded Language Models | Learning to Borrow -- Relation Representation for Without-Mention Entity-Pairs for Knowledge Graph Completion |
Character-based Neural Networks for Sentence Pair Modeling | Efficient Domain Adaptation of Sentence Embeddings Using Adapters | Non-Linguistic Supervision for Contrastive Learning of Sentence Embeddings |
Train Once, Test Anywhere: Zero-Shot Learning for Text Classification | Efficient Domain Adaptation of Sentence Embeddings Using Adapters | In Search for Linear Relations in Sentence Embedding Spaces |
Efficient Domain Adaptation of Sentence Embeddings Using Adapters | Clustering and Network Analysis for the Embedding Spaces of Sentences and Sub-Sentences | Character-based Neural Networks for Sentence Pair Modeling |
Hierarchical GPT with Congruent Transformers for Multi-Sentence Language Models | Learning Probabilistic Sentence Representations from Paraphrases | SentPWNet: A Unified Sentence Pair Weighting Network for Task-specific Sentence Embedding |
Sentence-T5: Scalable Sentence Encoders from Pre-trained Text-to-Text Models | Hierarchical GPT with Congruent Transformers for Multi-Sentence Language Models | Evaluating the Construct Validity of Text Embeddings with Application to Survey Questions |
In Search for Linear Relations in Sentence Embedding Spaces | Learning Joint Representations of Videos and Sentences with Web Image Search | Hierarchical GPT with Congruent Transformers for Multi-Sentence Language Models |
Learning to Borrow -- Relation Representation for Without-Mention Entity-Pairs for Knowledge Graph Completion | How to Probe Sentence Embeddings in Low-Resource Languages: On Structural Design Choices for Probing Task Evaluation | Learning Joint Representations of Videos and Sentences with Web Image Search |
考察
Amazon.Titan-Embed-Text-v1を使用する際、同じタイトルの文書が取得されました。この現象は、「chunking」と呼ばれる細分化して文書内でさらにどこが類似しているのかを検索できます。
llama_indexを使用し、chunkingとchunk_overlapを調整することで、検索する文章が細分化されます。
# BedrockとBedrockEmbeddingをllama_indexからインポートします from llama_index.llms import Bedrock from llama_index.embeddings import BedrockEmbedding # Titanモデルのパラメータを設定します model_kwargs_titan = { "stopSequences": [], "temperature":0.0, "topP":0.5 } # Bedrockのインスタンスを作成します llm = Bedrock( model="amazon.titan-text-express-v1", # amazon.titan-tg1-largeから変更 context_size=512, aws_region_name=region, additional_kwargs=model_kwargs_titan ) # BedrockEmbeddingのインスタンスを作成します embed_model = BedrockEmbedding().from_credentials( aws_profile=None, model_name='amazon.titan-embed-text-v1' # amazon.titan-embed-g1-text-02から変更 ) # チャンクのオーバーラップを設定します chunk_overlap = 20 # チャンクのサイズを設定します chunk_size = 512 # サービスコンテキストを設定します service_context = ServiceContext.from_defaults(llm=llm, embed_model=embed_model, chunk_size=chunk_size, chunk_overlap=chunk_overlap,) # グローバルサービスコンテキストを設定します set_global_service_context(service_context)
比較としてchunkingのサイズを拡大してみました。
Chunking=512 | Chunking=2048 |
---|---|
A Comparative Study of Sentence Embedding Models for Assessing Semantic Variation | A Comparative Study of Sentence Embedding Models for Assessing Semantic Variation |
Sentence-T5: Scalable Sentence Encoders from Pre-trained Text-to-Text Models | In Search for Linear Relations in Sentence Embedding Spaces |
In Search for Linear Relations in Sentence Embedding Spaces | Sentence-T5: Scalable Sentence Encoders from Pre-trained Text-to-Text Models |
Sentence-T5: Scalable Sentence Encoders from Pre-trained Text-to-Text Models | Are Classes Clusters? |
Are Classes Clusters? | Exploiting Twitter as Source of Large Corpora of Weakly Similar Pairs for Semantic Sentence Embeddings |
Are Classes Clusters? | SBERT studies Meaning Representations: Decomposing Sentence Embeddings into Explainable Semantic Features |
SBERT studies Meaning Representations: Decomposing Sentence Embeddings into Explainable Semantic Features | Vec2Sent: Probing Sentence Embeddings with Natural Language Generation |
SBERT studies Meaning Representations: Decomposing Sentence Embeddings into Explainable Semantic Features | Semantic Composition in Visually Grounded Language Models |
Exploiting Twitter as Source of Large Corpora of Weakly Similar Pairs for Semantic Sentence Embeddings | Efficient Domain Adaptation of Sentence Embeddings Using Adapters |
Vec2Sent: Probing Sentence Embeddings with Natural Language Generation | Clustering and Network Analysis for the Embedding Spaces of Sentences and Sub-Sentences |
Exploiting Twitter as Source of Large Corpora of Weakly Similar Pairs for Semantic Sentence Embeddings | Learning Probabilistic Sentence Representations from Paraphrases |
Semantic Composition in Visually Grounded Language Models | Learning Joint Representations of Videos and Sentences with Web Image Search |
Semantic Composition in Visually Grounded Language Models | How to Probe Sentence Embeddings in Low-Resource Languages: On Structural Design Choices for Probing Task Evaluation |
Efficient Domain Adaptation of Sentence Embeddings Using Adapters | Non-Linguistic Supervision for Contrastive Learning of Sentence Embeddings |
Efficient Domain Adaptation of Sentence Embeddings Using Adapters | Hierarchical GPT with Congruent Transformers for Multi-Sentence Language Models |
Clustering and Network Analysis for the Embedding Spaces of Sentences and Sub-Sentences | SentPWNet: A Unified Sentence Pair Weighting Network for Task-specific Sentence Embedding |
Learning Probabilistic Sentence Representations from Paraphrases | Character-based Neural Networks for Sentence Pair Modeling |
Hierarchical GPT with Congruent Transformers for Multi-Sentence Language Models | Train Once, Test Anywhere: Zero-Shot Learning for Text Classification |
Learning Joint Representations of Videos and Sentences with Web Image Search | Evaluating the Construct Validity of Text Embeddings with Application to Survey Questions |
How to Probe Sentence Embeddings in Low-Resource Languages: On Structural Design Choices for Probing Task Evaluation | Learning to Borrow -- Relation Representation for Without-Mention Entity-Pairs for Knowledge Graph Completion |
Chunkingのサイズを512から2048に変更したところ参照される文章が増え、詳細に検索されません。一部ランキングが変わりましたが、chunkingサイズを小さくすることでクエリに対して類似性の高い文章が検索できます。
OpenAIのText-Embedding-Ada-002は”Vec2Sent: Probing Sentence Embeddings with Natural Language Generation”が一番類似性がありました。AWSのBedrock Amazon.Titan-Embed-Text-v1は10番目にランク付されていました。
まとめ
検証の結果、異なるLLMを使用してクエリに対する文書の関連度を再ランク付けすることで、検索結果の順位が変わることが確認できました。チャットボットを運用する上で別のモデルに切り替えることで目的に応じた再ランクキングが可能で、検索の精度が上がることが期待できます。
今後に向けて
Re-rankingを用いて検索結果の順位が変わることが確認できましたが、本当に使えるものか検証する必要があります。以下が残課題として挙げられます。
- 定量的に分析ができていないため評価指数を設定して、それを用いて分析する。
- Reranking前と後でユーザーが必要な情報を得られたか、定性的に分析する。